GoGo Gadget: ChatPDF
Summarize the Research Paper "The false hope of current approaches to explainable artificial intelligence in health care"
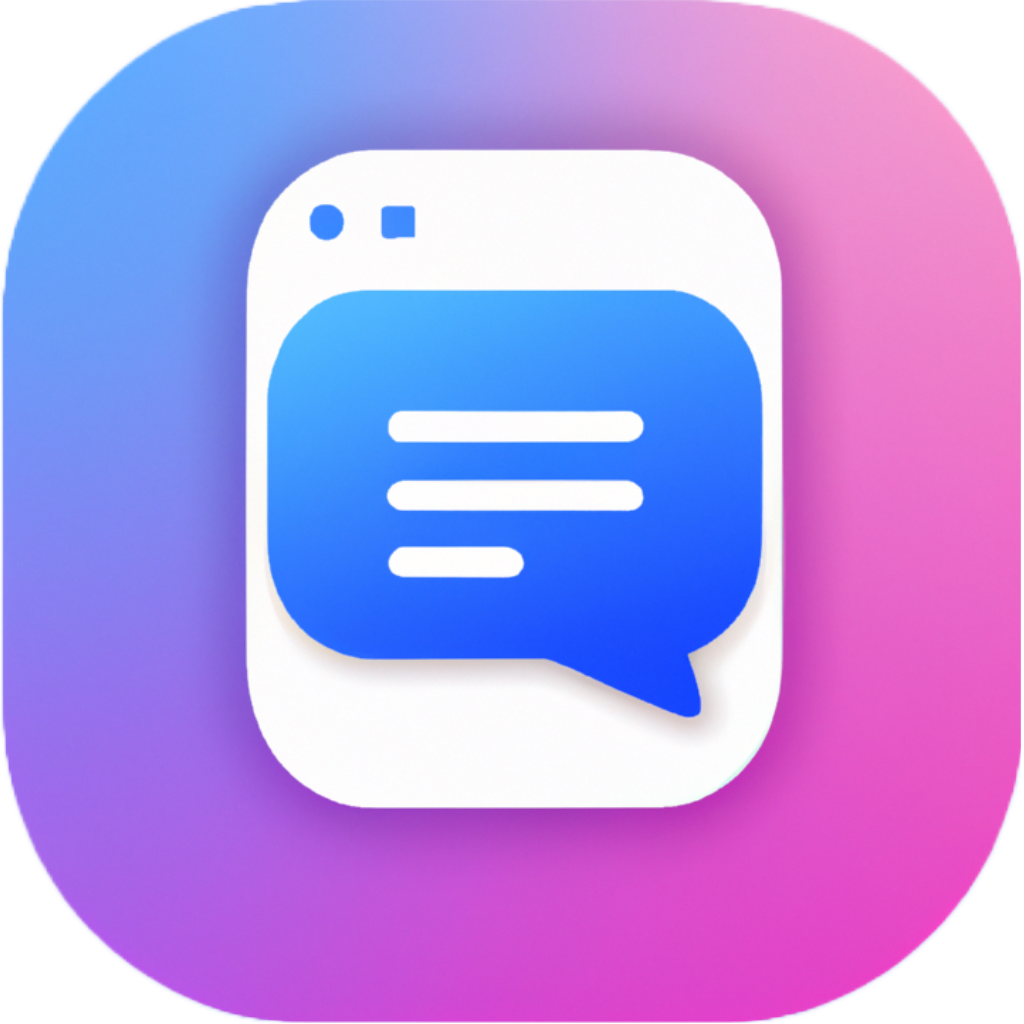
The research paper "The false hope of current approaches to explainable artificial intelligence in health care" argues that the current methods of explainable AI are unlikely to achieve the goals of patient-level decision support. The authors suggest that while AI will have a significant impact on medicine in the coming decades, it is important to ensure that this technology is implemented in a way that maximizes patient benefit. However, despite its intuitive appeal, explainability for patient-level decision making is unlikely to advance these goals in meaningful ways. The paper recommends that healthcare workers exercise appropriate caution when using explanations from an AI system and urges regulators to be judicious in listing explanations among the requirements needed for clinical deployment of AI.
Why this paper was selected
This paper has been selected due to following factors:
- The paper provides an overview of approaches towards explainable AI
- Health Care and Medicine are high-stake areas that can’t rely on approximations
- The authors outline possible failures of explainable AI approaches
- As a solution rigorous and thorough validation procedures are adviced
Explainability of individual or local decisions by AI
The authors discuss techniques for explainability that can generate general descriptions of how AI systems operate, but they note that these methods have limitations and can only offer unreliable or shallow explanations for specific decisions made by AI.
Inherent vs post-hoc explainability
Explainable AI approaches are categorized into two groups: inherent and post-hoc explainability:
Inherent explainability can be used for machine learning models that use a finite amount of input data with limited complexity in which the relatonship between input arguments can be clearly quantified and understood.
Post-hoc explainablity on the other hand is beeing used for modern AI systems that have high-dimensional and complex data and models in which the relationship between inputs and outputs cannot be quantified anymore.
Approaches towards AI explainability
For AI systems that give output on the input of images saliency maps can be used as a heatmap that reflect the degree of importance in an area on the image for the given output.
Interpretability gaps
The interpretability gap of explainability methods relies on humans to decide what a given explanation might mean. Unfortunately, the human tendency is to ascribe a positive interpretation: we assume that the feature we would find important is the one that was used (this is an example of a famously harmful cognitive error called confirmation bias). This problem is well summarised by computer scientist Cynthia Rudin: “You could have many explanations for what a complex model is doing. Do you just pick the one you ‘want’ to be correct?
Reasonability of decisions by AI
In the example of heat maps, the important question for users trying to understand an individual decision is not where the model was looking but instead whether it was reasonable that the model was looking in this region.
Sources
Ghassemi M., Oakden-Rayner L., Beam L. | The false hope of current approaches to explainable artificial intelligence in health care. The Lancet – 2019 |